The evolution of prosthetics has always been driven by a desire to restore not just movement, but the natural ease and responsiveness of the human body. While earlier designs focused on mechanical functionality, the advent of modern technologies has taken prosthetics into a new era. Among these advancements, machine learning stands out as a game-changer, enabling prosthetic devices to learn, adapt, and respond in ways that closely mimic the human body’s natural behaviors.
Machine learning is not just enhancing the technical capabilities of prosthetics—it’s transforming how users interact with their devices. By analyzing data, predicting user intentions, and adjusting in real-time, prosthetics powered by machine learning are setting new standards for performance and personalization.
The Role of Machine Learning in Prosthetics
Machine learning is a subset of artificial intelligence (AI) that enables systems to learn from data and improve their performance over time without explicit programming. In the context of prosthetics, this means creating devices that can adapt to the unique patterns, movements, and needs of individual users.
Traditional prosthetics rely on pre-programmed settings and fixed mechanical movements. While effective, they often fall short in dynamic environments or situations requiring fine motor skills.
Revolutionizing User Interaction
Machine learning transforms prosthetics into devices that actively learn from their users rather than requiring users to adapt to the device. For example, myoelectric prosthetics equipped with machine learning can interpret muscle signals more accurately, distinguishing between subtle variations in movements.
This allows users to perform complex tasks, such as typing on a keyboard or holding delicate objects, with precision and ease.
For businesses, investing in machine learning technologies that prioritize user comfort and adaptability can create a significant competitive edge.
Designing prosthetics that require minimal training or adjustment helps users integrate the device into their daily lives more seamlessly, fostering stronger brand loyalty and positive word-of-mouth.
Predictive Capabilities for Anticipating User Needs
One of the most groundbreaking aspects of machine learning in prosthetics is its ability to predict user intentions. By analyzing past behaviors, prosthetics can anticipate what a user is likely to do next and adjust their settings preemptively.
For example, a prosthetic leg might recognize that a user is about to transition from walking on flat ground to climbing stairs and adapt its resistance or angle accordingly.
This predictive capability reduces the cognitive effort required to operate the prosthetic, allowing users to focus on their activities rather than constantly managing their device.
For businesses, incorporating predictive algorithms into prosthetics provides a unique selling point, particularly for users seeking a more intuitive and responsive experience.
Enhancing Real-Time Responsiveness
In dynamic environments, the ability to respond quickly to changing conditions is critical for prosthetic performance. Machine learning enables prosthetics to process data from multiple sensors simultaneously, ensuring real-time responsiveness.
For example, a prosthetic foot can adjust its grip and balance when transitioning from dry to slippery surfaces, reducing the risk of falls and enhancing user confidence.
To optimize real-time performance, businesses should focus on integrating high-speed processing units and efficient algorithms into their prosthetic designs.
Collaborating with hardware manufacturers to develop lightweight yet powerful processors can ensure that prosthetics remain responsive without sacrificing comfort or battery life.
Supporting Long-Term Adaptation
Machine learning in prosthetics is not just about immediate functionality; it also supports long-term adaptation to the user’s evolving needs. Over time, a user’s physical condition, activity level, or preferences may change.
Machine-learning-enabled prosthetics can recognize these changes and adjust their behavior accordingly. For example, if a user begins engaging in more athletic activities, the prosthetic can adapt its settings to provide enhanced support and stability during high-impact movements.
For businesses, this adaptability translates into increased user satisfaction and prolonged device usage. Offering software updates or additional features through a connected app ensures that users can continue to benefit from the latest advancements without needing to replace their prosthetic entirely.
This approach not only adds value for customers but also creates opportunities for ongoing engagement and revenue generation.
Enhancing Precision and Responsiveness
One of the primary benefits of machine learning in prosthetics is its ability to enhance precision and responsiveness. This is achieved through the continuous processing of data collected by sensors embedded in the prosthetic device.
Real-Time Adjustments
Machine learning algorithms enable prosthetics to make real-time adjustments based on the user’s movements and environmental conditions.
For instance, an advanced prosthetic leg can detect changes in terrain—such as transitioning from flat ground to stairs—and automatically adjust its angle, stride length, or resistance to match the user’s needs.
This capability not only improves functionality but also reduces the risk of accidents or discomfort, particularly in unpredictable environments. For users, the prosthetic becomes an extension of their body rather than a tool they must consciously control.
Learning from User Behavior
Another critical aspect of machine learning is its ability to learn and adapt to individual users over time. By analyzing patterns in how a user moves, applies pressure, or interacts with their environment, the prosthetic can fine-tune its responses to match the user’s unique style and preferences.
For businesses, this opens up opportunities to create highly personalized prosthetic devices. By incorporating machine learning into their designs, manufacturers can deliver products that not only meet general functional requirements but also adapt to the nuances of each user’s lifestyle.
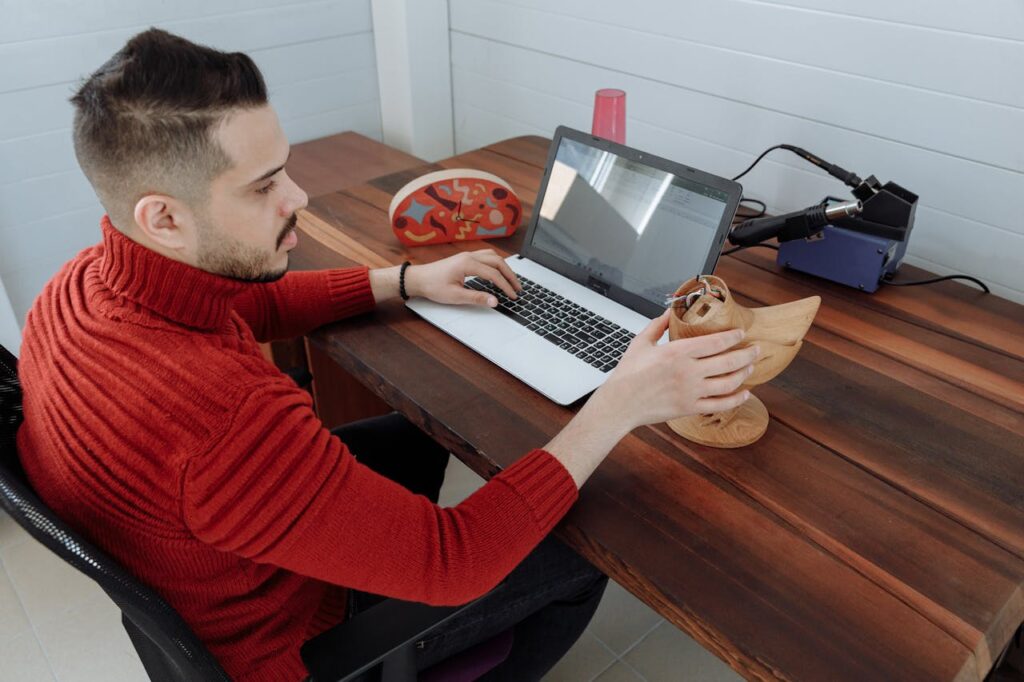
The Integration of Sensors and Machine Learning
The effectiveness of machine learning in prosthetics relies heavily on the integration of advanced sensors. These sensors collect critical data about the user’s movements, muscle activity, and surrounding environment, serving as the input for machine learning algorithms.
Types of Sensors Used
Different types of sensors are employed depending on the prosthetic’s purpose. Myoelectric sensors, for example, detect electrical signals generated by the user’s muscles, enabling fine control of prosthetic hands or arms.
Pressure sensors provide feedback on the amount of force being applied, ensuring delicate tasks—like holding a glass of water—can be performed without mishap.
For prosthetic legs, gyroscopes and accelerometers measure orientation and movement, allowing the device to maintain balance and adjust to changing surfaces.
These sensors, when paired with machine learning, create a system that not only reacts but anticipates the user’s needs.
Data Processing and Feedback Loops
The data collected by sensors is processed by the prosthetic’s onboard computer, which uses machine learning algorithms to analyze the information and generate appropriate responses. Feedback loops are integral to this process, enabling the prosthetic to continually refine its performance based on real-world usage.
For businesses, ensuring seamless integration between sensors, algorithms, and mechanical components is essential. This requires close collaboration between software engineers, hardware designers, and user experience specialists to create devices that are both functional and intuitive.
Personalization Through Machine Learning
Machine learning has redefined personalization in prosthetics, allowing devices to adapt to individual users in ways that were previously unimaginable.
By continuously analyzing and learning from user data, machine-learning-powered prosthetics can deliver a highly customized experience tailored to the unique preferences, habits, and needs of the wearer.
Adapting to Unique Movement Patterns
No two users move in exactly the same way. Machine learning enables prosthetics to understand and adapt to the subtle variations in an individual’s gait, grip strength, or motion preferences.
For example, a prosthetic leg can learn how a user typically walks, adjusts for asymmetries, and fine-tunes its performance to create a natural stride over time.
For upper-limb prosthetics, machine learning can identify patterns in muscle signals, allowing the device to interpret complex movements like rotating the wrist or varying grip strength for different objects.
This adaptation reduces the learning curve for users and makes the prosthetic feel more like a natural extension of their body.
Anticipating User Intentions
Advanced prosthetics equipped with machine learning can go beyond responding to commands—they can anticipate what the user intends to do next. By analyzing patterns in past behavior, these devices can predict actions and adjust their settings proactively.
For example, if a user frequently switches between walking and climbing stairs in a particular setting, the prosthetic leg can learn to anticipate these transitions and adjust its behavior seamlessly. This predictive capability enhances usability, making everyday tasks smoother and less taxing for the user.
For businesses, integrating anticipatory features into their products provides a significant competitive advantage, as it aligns with the growing demand for intuitive and user-friendly solutions.
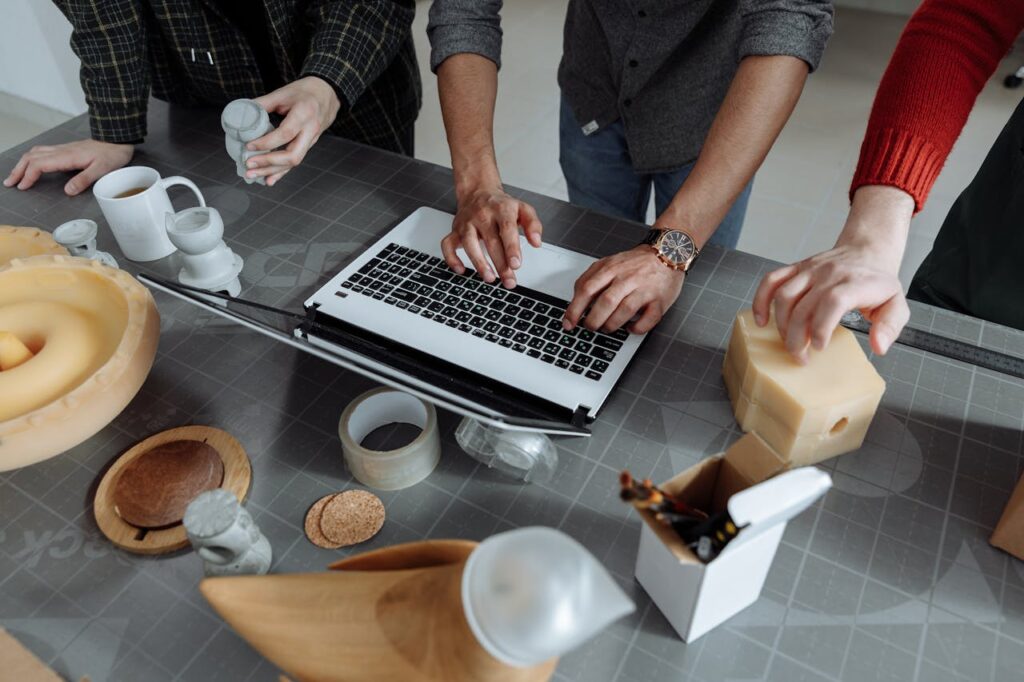
Improving Long-Term Performance
Machine learning offers significant advantages for the long-term performance and durability of prosthetics. Unlike traditional devices, which may require frequent manual adjustments or recalibration, machine-learning-enabled prosthetics continuously optimize themselves, ensuring consistent performance over time.
Continuous Learning and Improvement
One of the hallmarks of machine learning is its ability to evolve with the user. As the user’s needs or physical condition changes—such as gaining strength in certain muscles or adjusting to a new activity—the prosthetic can adapt accordingly.
This continuous learning process ensures that the prosthetic remains effective and comfortable, even years after it was first fitted. For businesses, this capability reduces the need for follow-up adjustments, saving time and resources while enhancing user satisfaction.
Remote Monitoring and Updates
Many modern prosthetics equipped with machine learning also feature connectivity options, allowing for remote monitoring and updates. Data collected from the prosthetic can be analyzed by healthcare providers or manufacturers, enabling proactive maintenance and performance optimization.
For example, if the prosthetic detects unusual patterns in movement or sensor performance, it can alert the user or their clinician to address potential issues before they escalate.
Businesses can leverage this feature to provide value-added services, such as periodic software updates or personalized coaching based on user data.
Expanding Accessibility Through Machine Learning
While machine learning brings advanced functionality to prosthetics, it also holds the potential to make these devices more accessible to a broader audience. Strategic implementation of this technology can help reduce costs, streamline production, and address the diverse needs of users.
Cost-Effective Manufacturing
Machine learning algorithms can simplify the customization process by automating key aspects of prosthetic design and fitting. For instance, data collected during initial assessments can be used to generate precise models tailored to the user’s anatomy and movement patterns.
This level of automation reduces the time and resources required for customization, making advanced prosthetics more affordable for users. For businesses, investing in machine-learning-driven design processes can improve efficiency while expanding access to high-quality devices.
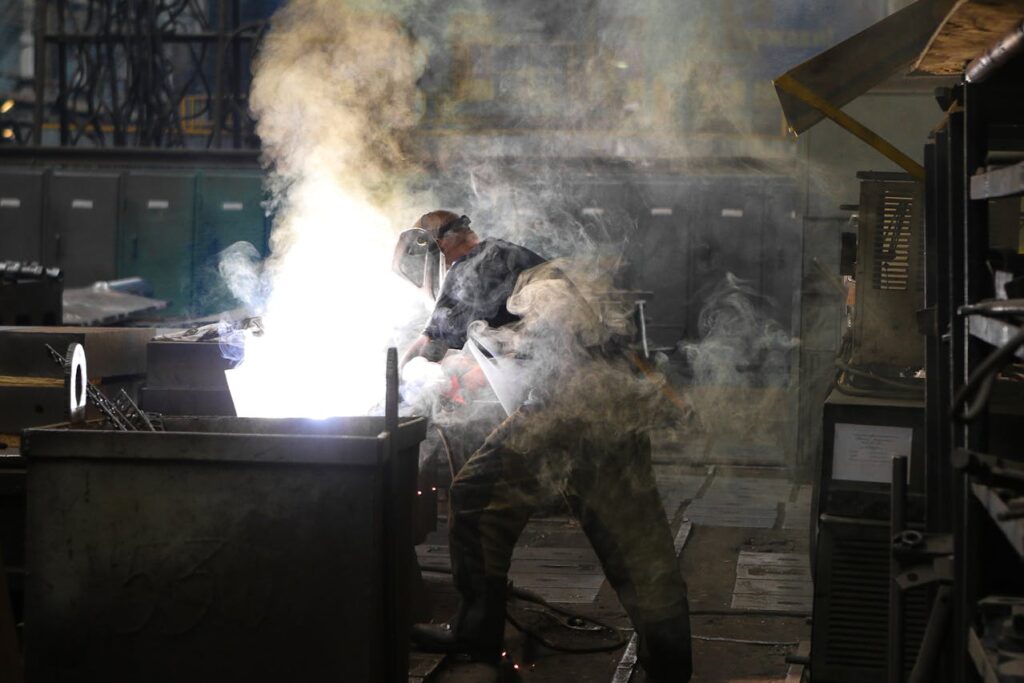
Bridging the Gap for Underserved Communities
Machine learning can also help address the unique challenges faced by users in underserved communities. For example, prosthetics equipped with self-learning capabilities require less frequent adjustments, making them ideal for users who have limited access to healthcare services.
Additionally, integrating multilingual interfaces or culturally relevant features into the prosthetic’s companion software can enhance usability for users in diverse regions.
Businesses that prioritize accessibility in their machine-learning solutions can establish themselves as leaders in inclusive innovation.
Future Trends in Machine Learning and Prosthetics
The potential of machine learning in prosthetics is still unfolding, with new advancements on the horizon that promise to further revolutionize the field. Emerging trends in neural integration, augmented reality, and advanced material science are set to push the boundaries of what prosthetics can achieve.
Neural Interfaces and Direct Communication
Neural interfaces are one of the most exciting developments in prosthetic technology. By connecting directly to the user’s nervous system, these interfaces enable seamless communication between the brain and the prosthetic.
Machine learning plays a crucial role in decoding neural signals and translating them into precise movements.
For businesses, investing in research and development for neural-integrated prosthetics represents a significant opportunity to stay at the forefront of innovation.
These devices not only enhance functionality but also create a deeper sense of connection between the user and their prosthetic.
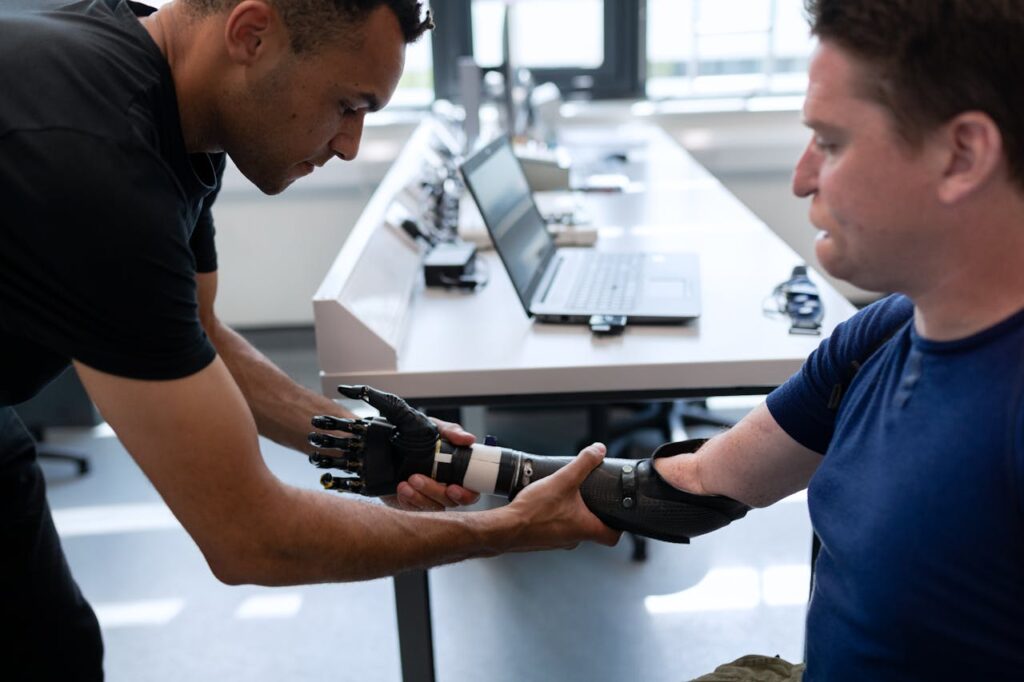
Enhanced Simulation and Training
Machine learning is also paving the way for enhanced simulation and training tools for prosthetic users. Augmented reality (AR) applications can simulate real-world scenarios, helping users learn how to interact with their prosthetics in a safe and controlled environment.
These tools can be particularly beneficial for first-time users, reducing anxiety and accelerating the adaptation process. For companies, offering AR-powered training programs alongside their prosthetics can add significant value and differentiate their products in the market.
Expanding Machine Learning Applications in Prosthetics
Machine learning is not only refining prosthetic performance but also redefining the ecosystem in which these devices operate. By integrating advanced capabilities like haptics, real-time diagnostics, and context-aware responsiveness, prosthetics are moving beyond mechanical aids to become intelligent companions. These advancements are creating new opportunities for businesses to innovate and meet the evolving demands of users.
Incorporating Haptic Feedback
Haptic feedback is a transformative feature that provides users with a sense of touch through their prosthetics. Machine learning enhances this capability by processing sensor data and delivering nuanced feedback that mimics the sensations of texture, pressure, or temperature.
For instance, a prosthetic hand equipped with haptic technology can allow a user to differentiate between smooth and rough surfaces or gauge the firmness of their grip. Machine learning algorithms analyze and refine the feedback based on the user’s preferences, making the sensations more accurate and intuitive over time.
Businesses can capitalize on this by developing prosthetics that prioritize sensory realism, providing users with a deeper connection to their environment. Highlighting these capabilities in marketing materials can appeal to users seeking advanced functionality and emotional engagement.
Context-Aware Prosthetic Behavior
Machine learning enables prosthetics to become context-aware, adjusting their behavior based on environmental cues and user activity. For example, a prosthetic leg can recognize when the user is transitioning from walking to running and modify its joint resistance accordingly.
Similarly, a prosthetic arm can adapt its grip strength based on the weight and texture of the object being held.
This adaptability reduces the cognitive load on users, allowing them to focus on their tasks rather than constantly adjusting their prosthetic. For businesses, integrating context-aware features into their products creates a compelling value proposition, particularly for active users or those engaged in diverse activities.
Real-Time Diagnostics and Predictive Maintenance
Machine learning’s ability to analyze data in real-time also enhances the diagnostic and maintenance aspects of prosthetic devices. Sensors embedded in the prosthetic continuously monitor performance metrics, such as joint movement, battery levels, and wear on mechanical components.
If the system detects anomalies—such as increased resistance in a joint or irregular sensor readings—it can alert the user or their healthcare provider. Predictive maintenance capabilities allow potential issues to be addressed proactively, reducing downtime and extending the prosthetic’s lifespan.
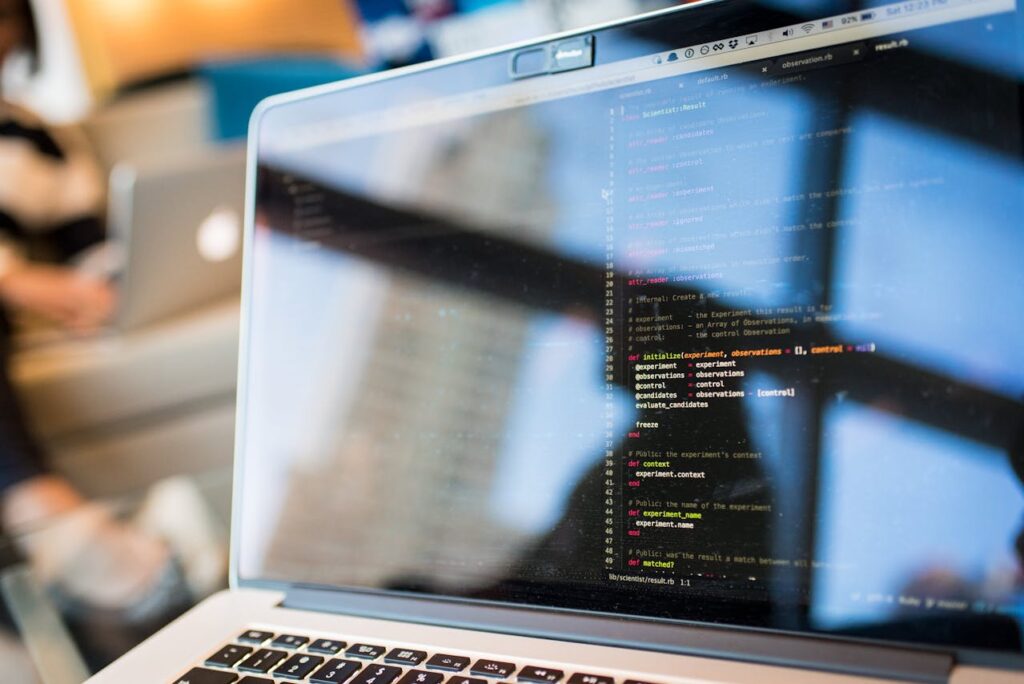
For businesses, offering diagnostic and maintenance features not only improves the user experience but also opens up opportunities for after-sales services, such as maintenance packages or subscription plans for software updates.
Addressing Ethical Considerations in Machine Learning for Prosthetics
As machine learning becomes more deeply integrated into prosthetics, it raises important ethical considerations that businesses must address. These include data privacy, user autonomy, and the equitable distribution of advanced technologies.
Ensuring Data Privacy
Machine-learning-enabled prosthetics rely on continuous data collection to refine their performance. This includes sensitive information such as muscle signals, movement patterns, and usage habits.
Businesses must implement robust data privacy measures to protect users’ personal information and comply with regulations like GDPR or HIPAA.
Providing users with transparency about how their data is collected, stored, and used is critical. Companies can enhance trust by offering opt-in data-sharing options and ensuring that all data is anonymized when used for research or product development.
Empowering User Autonomy
While machine learning enhances prosthetic performance, it’s essential that users retain control over their devices. Businesses should prioritize user-centric designs that allow individuals to customize settings, override automated behaviors, and understand how their prosthetic operates.
For instance, providing a companion app that enables users to adjust parameters—such as haptic feedback intensity or movement sensitivity—ensures that the prosthetic aligns with their preferences.
By empowering users to make decisions about their prosthetic’s functionality, companies can create products that feel truly personalized.
Expanding Access to Advanced Technologies
One of the challenges in integrating machine learning into prosthetics is ensuring that these advanced devices are accessible to users across different economic backgrounds and regions. Businesses must balance the cost of innovation with the need for affordability and inclusivity.
Collaborating with government programs, NGOs, and healthcare providers can help subsidize the cost of machine-learning-enabled prosthetics for underserved populations.
Additionally, offering tiered pricing models—where users can choose from basic to premium features—ensures that a wider audience can benefit from this technology.
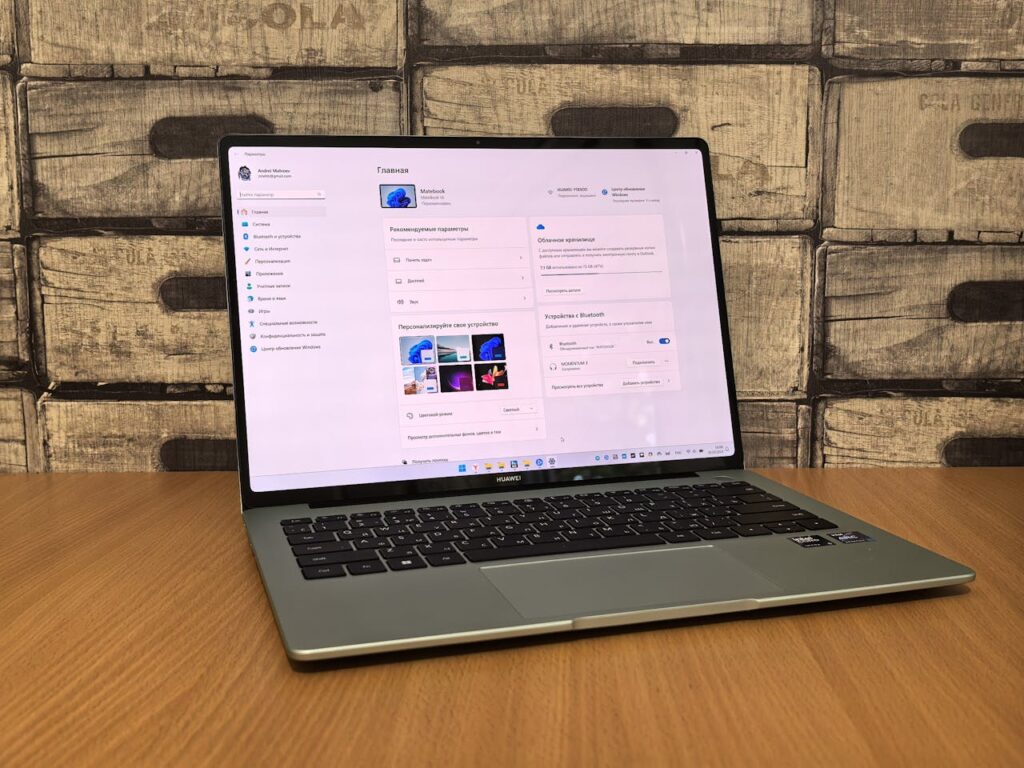
Looking Ahead: The Future of Machine Learning in Prosthetics
The future of prosthetics lies in the seamless integration of machine learning with other cutting-edge technologies. Developments in neural interfaces, augmented reality, and biocompatible materials are set to revolutionize how prosthetics interact with the human body and the environment.
Neural Interfaces and Direct Brain Control
Neural interfaces are becoming increasingly viable, allowing prosthetics to be controlled directly by brain signals. Machine learning plays a crucial role in decoding these complex signals, translating them into precise movements.
As these interfaces become more advanced, they will enable prosthetics to feel even more natural, reducing the gap between biological and artificial limbs.
For businesses, investing in research and development for neural-integrated prosthetics positions them at the forefront of this transformative field.
Collaborative Ecosystems
The future of prosthetics will also involve greater collaboration between users, manufacturers, and healthcare providers. Machine-learning-enabled prosthetics can serve as a hub for communication, sharing data with clinicians to improve rehabilitation outcomes and providing feedback to manufacturers for continuous product improvement.
By fostering these collaborative ecosystems, businesses can create holistic solutions that go beyond the prosthetic itself, delivering comprehensive support and care to users.
Conclusion
Machine learning is revolutionizing prosthetic performance, creating devices that are not only functional but also intuitive, adaptive, and deeply personalized.
By leveraging real-time data analysis, predictive algorithms, and advanced sensor integration, machine-learning-enabled prosthetics are bridging the gap between technology and the human body.
These innovations empower users to live more confidently, perform tasks with greater precision, and experience a seamless connection with their prosthetic devices.
For businesses, the opportunities are immense. From enhancing personalization to integrating context-aware features, machine learning offers a pathway to deliver prosthetics that meet the diverse and evolving needs of users.
By prioritizing accessibility, data privacy, and ethical considerations, companies can ensure that their innovations reach a broader audience while maintaining user trust and satisfaction.